The artificial intelligence (AI) algorithm that powers Genderfluent’s gender estimation is called an artificial neural network, which is commonly referred to as “deep learning” in the AI community. The way the algorithm works is inspired by how the human brain works, where you have many neurons and there are many connections between them. These algorithms have turned out to be extremely powerful and have exploded in popularity over the past ten years. Today, they power everything from language translation, cancer diagnosis, voice recognition, and self driving cars. And we at Genderfluent thought it seemed obvious that AI should also help the transgender community. 😊
How we applied AI to estimate gender
We spent a lot of time developing our AI algorithm to estimate gender. While other individuals and researchers in the past have create such algorithms, we found they underperform when applied to transgender voices. The vocal characteristics of transgender people who are actively training their voice present unique challenges that are typically not seen in the much more common cis-male and cis-female voices. The main example to give here is pitch.
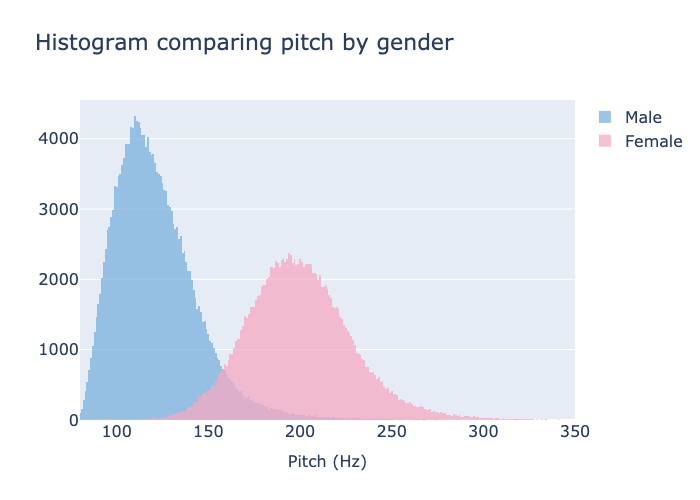
Pitch is the perceived “highness” or “lowness” of a voice. Males have a lower pitch typically between 80Hz and 180Hz, while females have a higher pitch typically in the range of 155Hz to 255Hz. Anatomically, pitch is the frequency at which your vocal folds vibrate. Counterintuitively, pitch actually plays a secondary role in the perceived gender of a voice. While it certainly can help make your voice sound more like your target gender, it is not the most important factor. Unfortunately, many voice training therapists, guides, and software apps put too much emphasis on needing to change pitch. So this is where AI comes into the picture. One of the main challenges we faced when training a gender classifier is getting our algorithm to downplay the importance of pitch.
We collected hundreds of hours of recorded audio from both male and female speakers. Importantly, this dataset includes many voices whose pitch is uncommon for their gender (i.e. a cis-female with low pitch and cis-male with high pitch). This diverse dataset is what enables us to more robustly estimate gender.
Does it really work?
Yes! We achieved over 98% accuracy when predicting gender over an audio clip. To showcase this, below are some 10-second audio clips with estimated gender and pitch for various speakers. The plot with pink and blue boxes shows the estimated female- or male-ness, respectively, of each 1 second window of audio. If it shows mostly pink boxes, then the speaker is estimated to be female. Similarly, if mostly blue boxes, then the speaker is estimated to be male.
Example 1: cis female voice.
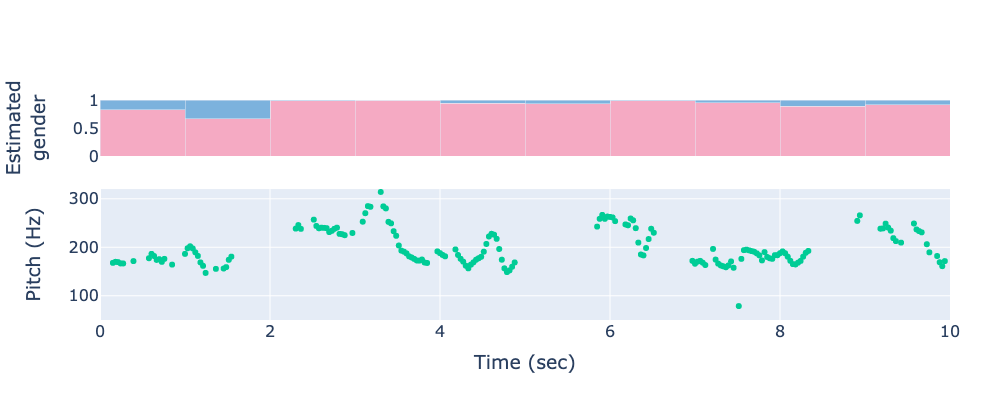
Example 2: cis male voice.
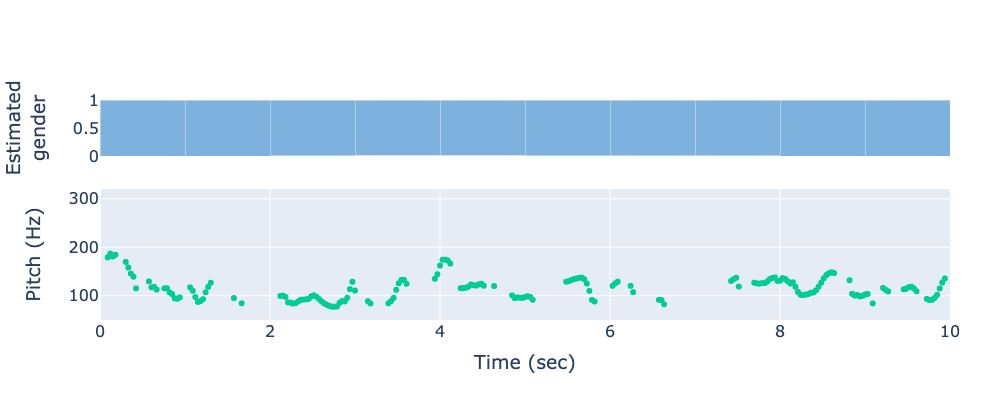
Example 3: Shohreh Aghdashloo, who is an actress with a famously deep voice.
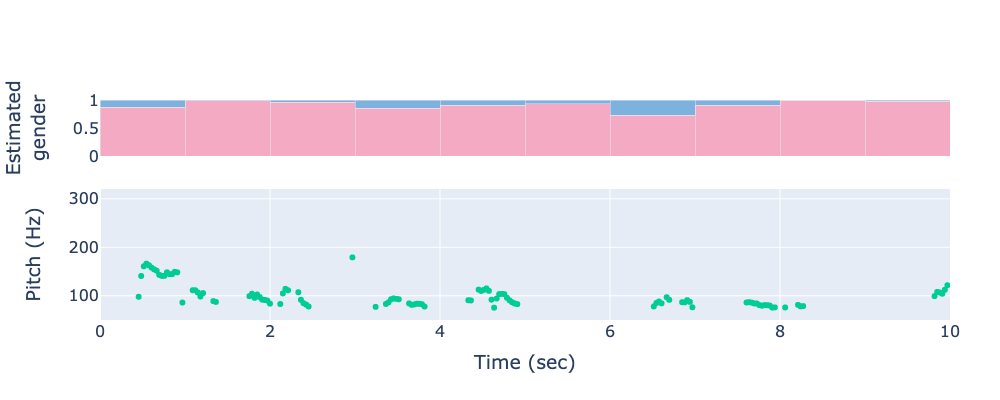
Example 4: A YouTuber (Chef John) whose voice is high pitched for a male.
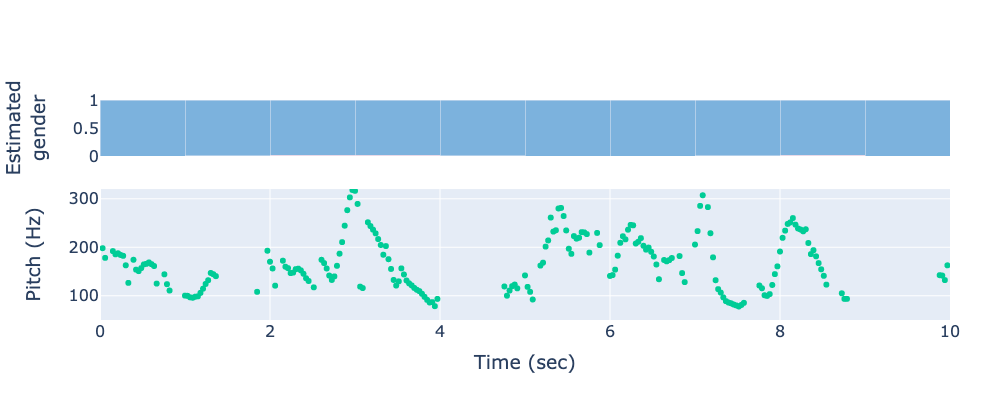
Example 5: A somewhat androgynous-sounding voice.
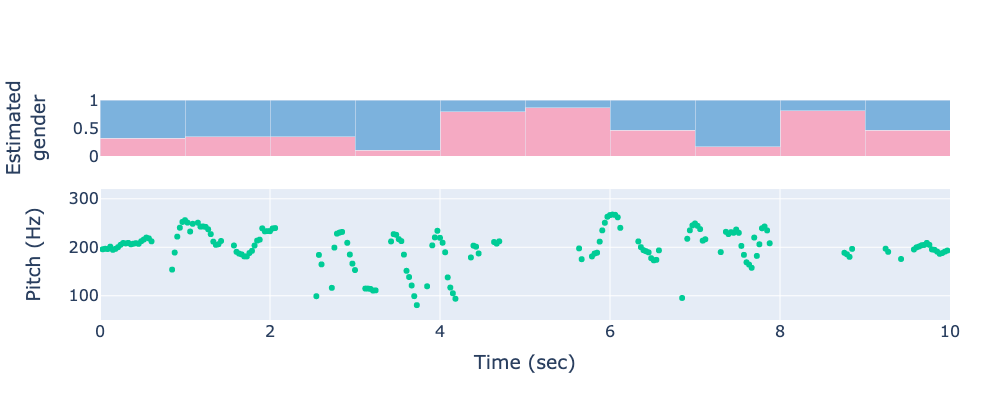
Final remarks
We’re pleased with how our AI algorithm is performing, but as with any software, it may not perform optimally in every situation. Factors such as microphone quality, background noise (e.g. fans, music), spoken language, age, etc. can all potentially impact the performance of the AI algorithm. Therefore, with help from users of Genderfluent, we aim to continuously make improvements over time.